By Vishal Goel, | December 27, 2016
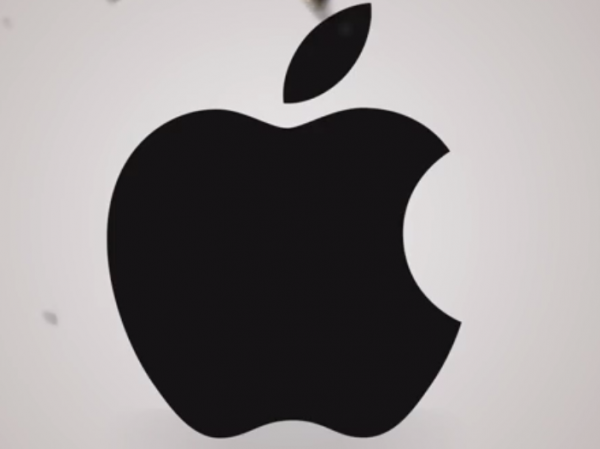
Apple is proposing to use modified Generative Adversarial Networks (GANs) to improve the quality of these synthetic training images. (YouTube)
Six researchers working for tech giant Apple have published a paper describing a novel method of simulated and unsupervised learning with the aim of improving the quality of synthetic training images.
Earlier this month, Apple's Director of AI Research, Russ Salakhutdinov, announced that the company was getting into publishing research. The six researchers who published the paper belong to a recently formed machine learning group.
Like Us on Facebook
Synthetic images and videos are being used to train machine learning models. They are readily available, less costly, and customizable. Although the new technique reportedly has a lot of potential, it is risky because generated images do not meet the same quality standards as real images.
Apple is proposing to use modified Generative Adversarial Networks (GANs) to improve the quality of these synthetic training images. In a nutshell, GANs work by taking advantage of the adversarial relationship between competing neural networks. In Apple's case, the simulator-generated synthetic images are run through a refiner and then sent to a discriminator that is tasked with distinguishing real images from synthetic ones.
Apple's variation of GANs tries to minimize both local adversarial loss and self-regulation. These terms simultaneously minimize the differences between synthetic and real images while minimizing the difference between synthetic and refined images to retain annotations. The idea here is that too much alteration can destroy the value of unsupervised training.
The research paper titled "Learning from Simulated and Unsupervised Images through Adversarial Training" can be accessed here.
-
Use of Coronavirus Pandemic Drones Raises Privacy Concerns: Drones Spread Fear, Local Officials Say
-
Coronavirus Hampers The Delivery Of Lockheed Martin F-35 Stealth Fighters For 2020
-
Instagram Speeds Up Plans to Add Account Memorialization Feature Due to COVID-19 Deaths
-
NASA: Perseverance Plans to Bring 'Mars Rock' to Earth in 2031
-
600 Dead And 3,000 In The Hospital as Iranians Believed Drinking High-Concentrations of Alcohol Can Cure The Coronavirus
-
600 Dead And 3,000 In The Hospital as Iranians Believed Drinking High-Concentrations of Alcohol Can Cure The Coronavirus
-
COVID-19: Doctors, Nurses Use Virtual Reality to Learn New Skills in Treating Coronavirus Patients